Drug Maps and Idea Paths
All models are flawed and cows are not perfect spheres, but that doesn't mean we can't build better maps
In today’s idea mix:
A drug discovery GPS
The route from strange Babylonian stars to Newton’s universal gravitation
All models are flawed and your brain is weird
Building a GPS for drug discovery
Let’s start with a few numbers. Don’t worry, there’s a point, I promise.
It takes on average 10-15 years and $1.5–2.5 billion to develop an FDA-approved drug, and 90% of clinical drug development fails. The main reasons for failure are lack of clinical efficacy (40%–50%), unmanageable toxicity (30%), poor drug-like properties (10%–15%), and lack of commercial needs plus poor strategic planning (10%).
Modern drug development summarized: Throw stuff at the wall and hope something sticks1.
So why not build a GPS for drug discovery? That's the idea proposed in a short new paper. When considering the high rates of drug development failure, the authors write:
There has never been a better time to fix this: the explosion of biomedical data of millions of humans linked with genetic information and clinical outcomes…
Okay, okay, but what does that mean? It means we have more information to build a map with routes from problem to treatment. Here be dragons. I mean, drugs.
Disciplines such as proteomics (measure all the proteins!) and metabolomics (all the molecules involved in metabolism!) lead to a more detailed understanding of human physiology and can help us identify biomarkers. Add genetics via high-throughput sequencing (collecting a lot of information quickly) and genome-wide association studies (finding specific genetic 'signals' in a population) and the map resolves a little more. Sprinkle some machine learning over it, and - presto - a map with potential routes from disease problem to druggable solution. Don’t tell anyone, but this is a strategy many healthcare startups are pursuing.
This is not a fix-it-all approach and drugs are certainly not always the best answer to health challenges. But companies that include human evidence (genetics, biomarkers) to guide drug discovery have a 2.6-fold higher success rate than the industry standard.
Turn on the GPS.
The path from ancient Babylon to Newton
Why stick to drugs and molecules, though? Can we do the same with, say, the development of ideas?
That’s the idea (couldn’t resist, sorry) behind something I stumbled on recently: the path to Newton — an effort by the Prediction project led by Harvard Professor Alyssa Goodman to map the historical ideas that were combined and refined to culminate in Newton’s insights on the role of gravity in celestial motion.
Start with ancient Babylonian astronomical diaries that note how some stars moved differently from others, travel along ancient Greeks and Romans who tried to understand nature, add fancy mathematics from Middle and Far Eastern sources, spice it up with observations by Copernicus and Kepler, and… welcome to Newton’s ‘gravity makes the planets go round and round’.
Here is the starting point of the map (probably too small in email, use the link in the caption for a zoomable version).
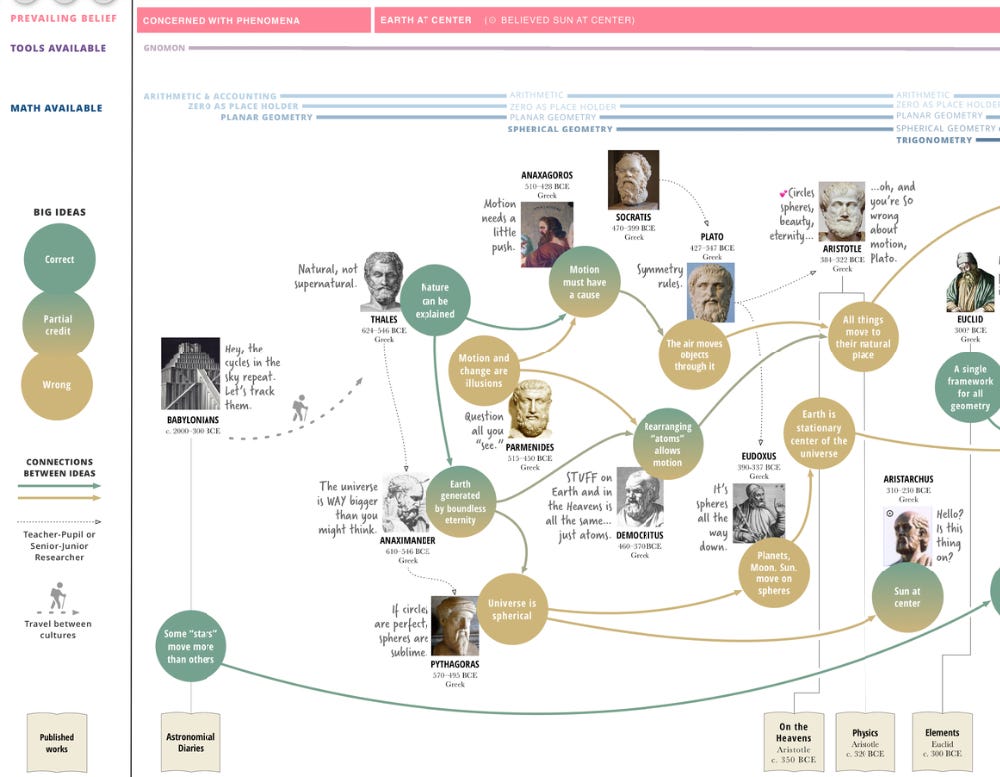
Flawed models, giant maps, and spherical cows
The map, however, is never the territory.
A famous one-paragraph story by Jorge Luis Borges2, On Exactitude in Science, illustrates this by imagining an empire that wanted a perfect map of its territory. Eventually, the map became as large as the empire itself.
Any map, or any scientific model, is subject to two processes: abstraction and idealization3. Abstraction: take out what you consider ‘not relevant enough’; idealization: specify specific (‘ideal’) conditions.
This is where I need to introduce spherical cows. The spherical cow metaphor is a humorous indictment of oversimplification in scientific models. A short version:
A farmer was struggling with the milk production on his dairy farm, so he asked a physicist for help. After the physicist worked through the problem, he told the farmer, "I have the solution, but it only works for spherical cows in a vacuum."
Abstraction: the farm environment is removed. Idealization: the cow is modeled as a perfect sphere.
Of course, few scientific models are simplified to this extent, but they are still simplified. An example from my biology days is the Hardy-Weinberg equilibrium, which helps us quantify the frequencies of gene variants (alleles). This model makes several abstractions (no selection, for example) and idealizations (infinite population size, for example). Not a single population of organisms adheres to the seven key assumptions of the Hardy-Weinberg equilibrium. But that doesn’t mean the model is pointless.
No, my point (badum tss) is that we can learn a lot from models by understanding when, how, and why reality deviates from what the models tell us. Sometimes, the drug GPS will steer us in the wrong direction, no matter how much data and machine learning we throw at it. Why? Because biology is both messy and fuzzy. Sometimes, idea graphs will have holes. Why? Because not every idea along the way has been documented or attributed (especially female contributions have a long history of being erased, which is a well-documented phenomenon known as the Matilda effect).
Let’s take it up a notch.
Your brain spends a lot of time and energy building mental models — internal representations of external reality. For example, photons splash onto your eyes, are focused by the lens, travel through the inner eye blob that we call the vitreous body, and then activate rod and cone cells in the retina. Based on that input, your brain constructs shapes, edges, and colors. To do that, your brain abstracts (filters out sensory noise) and idealizes (perceptual constancy, such as recognizing the same color in different lighting conditions).
Uh-oh. If all models are flawed and our experience of reality is mediated through mental models…
Because I footnoted Lewis Carroll, I have to end with this quote:
I am not crazy, my reality is just different from yours.
— Cheshire Cat
90% sarcasm.
He based it on an idea from Lewis Carroll’s Sylvie and Bruno Concluded.
This is a point I recently came across in The Brain Abstracted by Mazviita Chirimuuta, a professor of history and philosophy of science. In the book, Chirimuuta looks at simplification in neuroscience and how this has shaped our understanding of the brain (as well as why the computational view of our white-and-gray matter might not be an accurate representation of reality).
The last quote of the Cheshire Cat suits you perfectly.